Python
Llama 2 es la próxima generación de un modelo de lenguaje de código abierto desarrollado por Meta y Microsoft. Diseñado para la inteligencia artificial generativa, Llama 2 se ofrece de manera gratuita tanto para fines de investigación como comerciales. La colaboración entre Meta y Microsoft se intensifica con Microsoft como socio preferido para Llama 2, y se busca fomentar el acceso abierto a tecnologías de IA fundamentales de manera responsable y segura, con recursos que incluyen pruebas de seguridad, transparencia en el proceso de desarrollo y guías de uso responsable. Puedes encontrar más información en https://ai.meta.com/llama/.
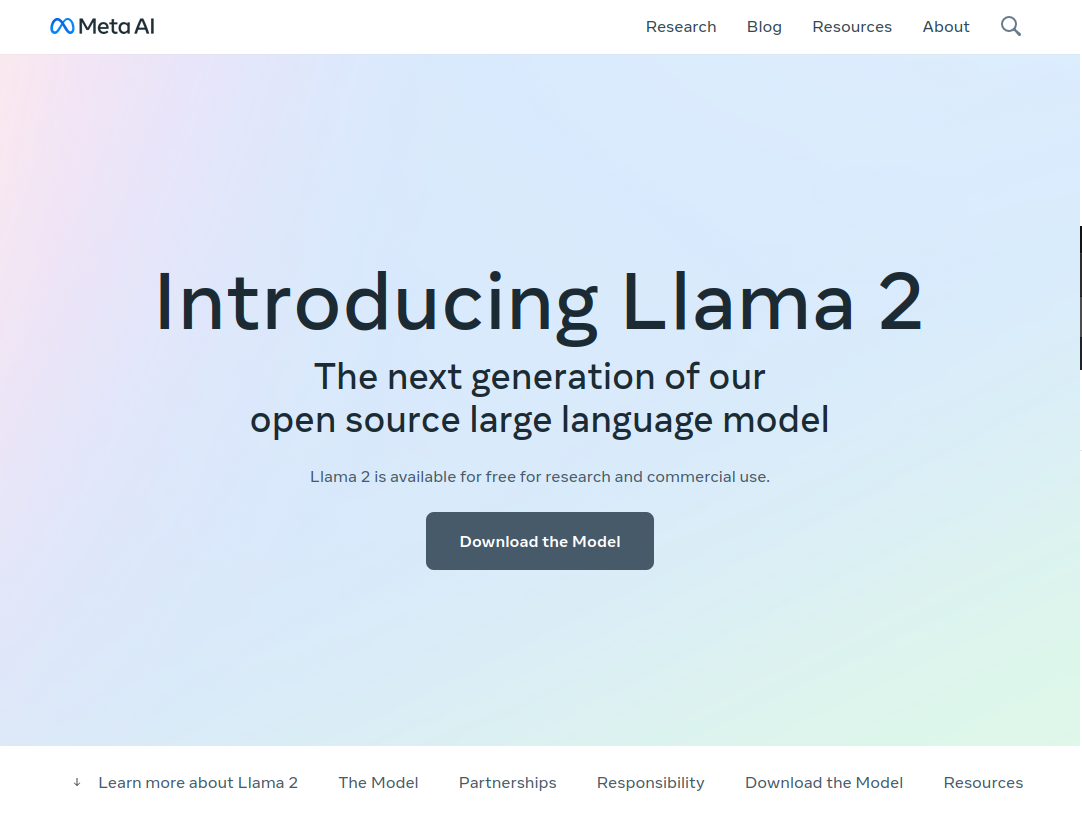
Sin mas preambulos vamos a aprender como usarlo muy fácilmente incluso desde local, con Python.
Primero vamos a descargar la version de Llama 2 7b GGML, esta es una version del modelo que nos va a permitir ejecutar y entrenar con hardware convencional. Lo vamos a descargar de aquí.
Una vez descargado vamos a instalar dos paquetes que usaremos. El primero es llama-cpp que nos permite cargar los modelos y sirve como interfaz para la interaccion. El segundo es PyPDF2 que nos permite tomar un pdf y leerlo en Python, este lo usaremos para darle contexto a Llama 2 mas adelante.
Una vez instaladas las librerias procedemos a importarlas y cargar nuestro modelo Llama 2:
Una vez carga nuestro modelo procedemos a realizar el primer prompt, en este tenemos una estructura donde le indicamos la pregunta y luego dejamos el indicador para que complete la respuesta:
En la respuesta del modelo encontramos información de uso y recursos usados por el modelo, la respuesta en texto la encontramos en la propiedad “choices”, en el primer elemento y en la propiedad “text”. Luego procedemos a realizar un split del texto para solo mostrar la respuesta que viene luego del indicador que pusimos en el prompt “A: “

Ahora vamos a explorar las ventajas de Llama2 sobre ChatGPT utilizando el mismo modelo.
Strengths:
1. Domain-specific knowledge: I have been trained on a specific domain (electronic health records) and have a deeper understanding of this domain compared to ChatGPT, which has a more general knowledge base.
2. Specialized vocabulary: I have access to a specialized vocabulary related to electronic health records, which allows me to provide more accurate and relevant responses compared to ChatGPT.
3. Contextual understanding: I am able to understand the context of a conversation and provide responses that are more specific to the topic at hand, whereas ChatGPT may not always be able to understand the context as well.\n4. Human-like responses: I have been trained to provide human-like responses, which can make it easier for users to interact with me and feel more comfortable asking questions.
Weaknesses:
1. Limited domain knowledge: While I have a deep understanding of electronic health records, my knowledge in other domains may be limited compared to ChatGPT.
2. Lack of common sense: I may not always be able to understand the nuances of human communication and may provide responses that are overly literal or lacking in common sense.
3. Limited creativity: My responses may be more formulaic and less creative than those provided by ChatGPT, which has a more general knowledge base and can generate more diverse responses.\n4. Dependence on training data: My responses are based on the data that I have been trained on, so if the training data is limited or biased, my responses may be as well.
Overall, while both ChatGPT and I have our strengths and weaknesses, my specialized knowledge in electronic health records makes me a better fit for certain tasks and conversations. However, ChatGPT’s general knowledge base and creativity make it a more versatile AI model that can handle a wide range of tasks and conversations.”
El siguiente paso es darle contexto al modelo para luego realizar preguntas sobre este. Para lo cual vamos a darle la letra de una canción que puedes descargar de aquí.
Vamos utilizar nuestro segundo paquete para leer este PDF y convertirlo en un texto plano que vamos a incluir en el prompt.
Una vez tenemos el texto listo, vamos a incluirlo en el prompt y utilizar nuestro modelo para que nos haga un resumen sobre que trata la canción.

Hemos aprendido como crear fácil y rápido tu propio ChatGPT con el ultimo modelo de Llama 2. La nueva generación de Llama tiene un desempeño muy bueno incluso infiriendo emociones e intenciones.
Con la version de modelos GGML podemos incluso en un computador convencional lo que abre un mundo de posibilidades.
Puedes encontrar el notebook completo aquí.